Insights
- category:
How to keep privacy in the age of Big Data?
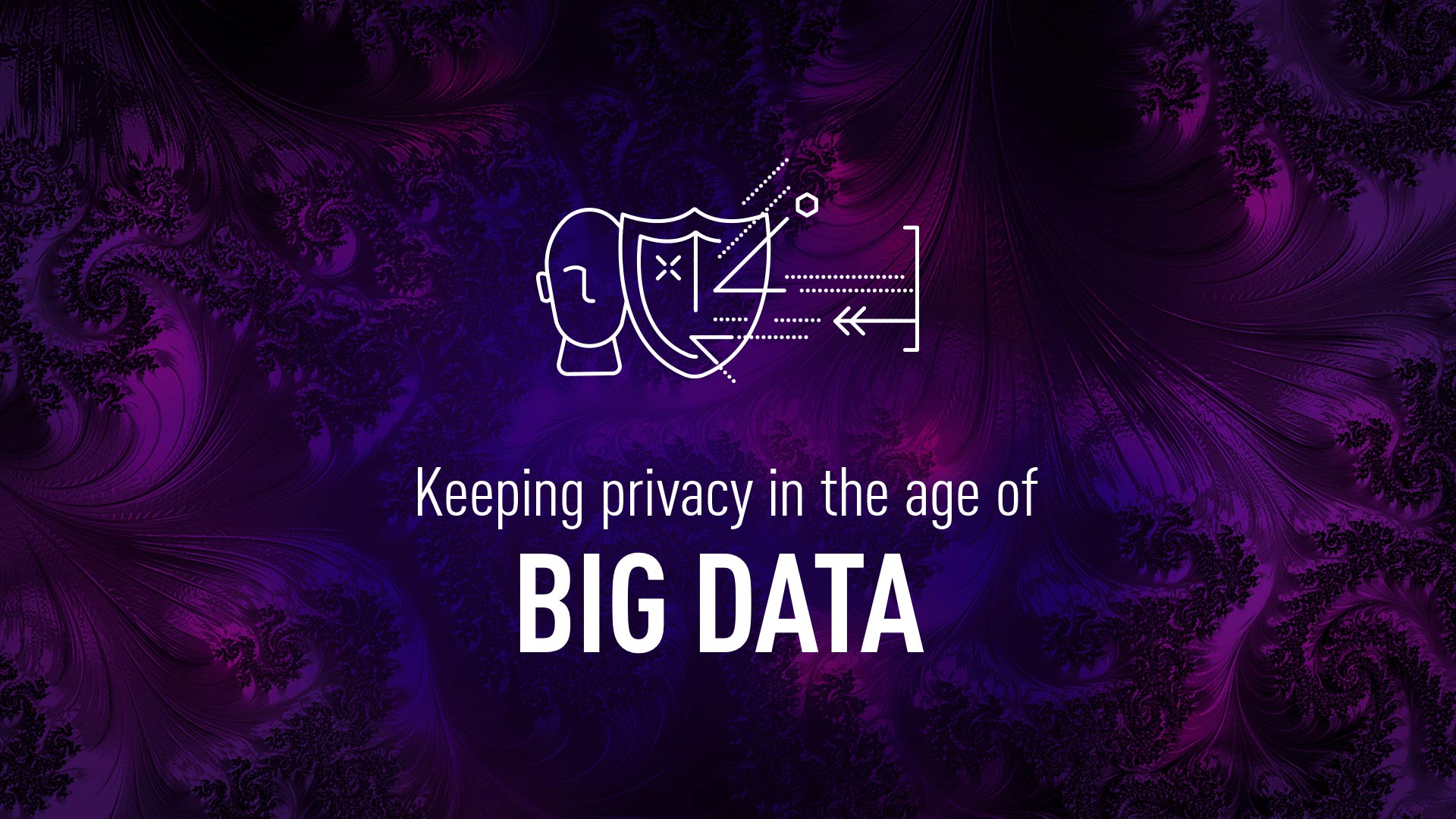
Poor management of Big Data is a real privacy risk. But without data analytics, companies have a hard time understanding customers’ behaviours and making smart decisions. That’s why a carefully planned data management strategy is crucial to ensure that data moves around with minimal risk. But how can we make sure to keep privacy in the age of Big Data?
Main Big Data security risks
Big Data analytics provides insights about people that are far and above what they know about themselves. And that is a big responsibility, especially when talking about Big Data privacy. The problem of our data security existed before, but until Big Data analytics showed us the tools to be highly accurate with various predictions, we weren’t that scared.
Big Data offers many benefits to companies, but there are a few privacy risks that we should think about when using analytics tools:
- Data breaches – when information is accessed without authorization. These mostly happened because of out-of-date software, weak passwords, or targeted malware attacks. Such breaches can cost a company lots of money and damage its reputation. That’s why companies should keep their software up to date, change passwords often, and focus on educating employees about security.
- Data brokerage – when unprotected and incorrect data is sold. Some companies gather and sell customer profiles which contain false information that leads to flawed algorithms. Before buying data, companies should do their research to make sure they are receiving data from a reputable provider that offers accurate data.
- Data discrimination – when some algorithms penalize individuals from particular data. Since data can consist of customer demographic information, companies may develop algorithms that penalize individuals based on age, gender, or ethnicity. They should always have a thorough and accurate representation of customers, account for biases, and put fairness above analytics.
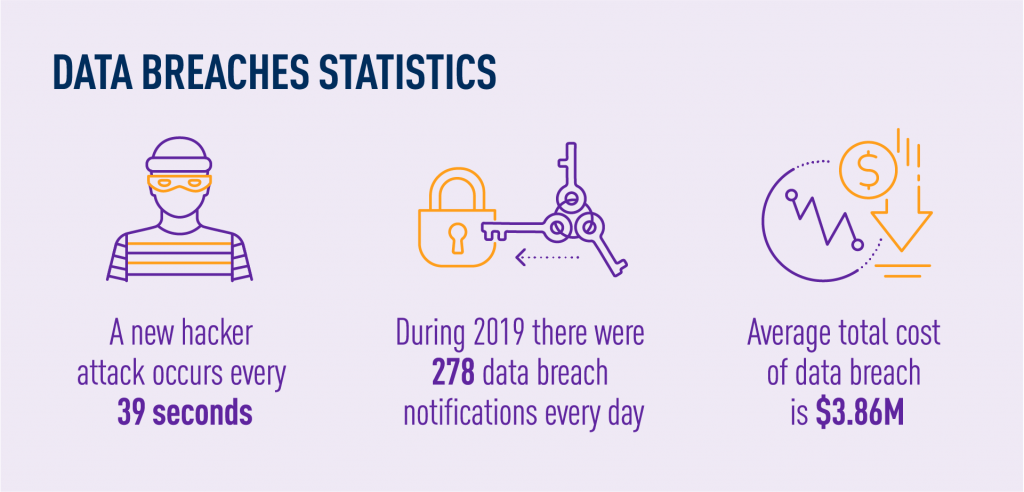
When most people talk about privacy in the age of Big Data, their biggest concerns are connected with their personal data. They know that their account numbers or other sensitive information are out there, and if a breach occurs, it will have unpleasant consequences. They are also scared of the information that is gathered to understand our needs. We want to receive personalized offers and suggestions, but when we think closer about that, it might scare us. That’s when another level of our concern shows up – knowledge about what these Big Data tools actually know. And it concerns so many areas of our lives.
Analytics techniques for ensuring Big Data privacy
As there is an increasing need for solving the challenges of security and privacy, we wanted to introduce some techniques that help with keeping privacy in the age of Big Data – a few methods of cryptography and anonymous protection techniques in the social network.
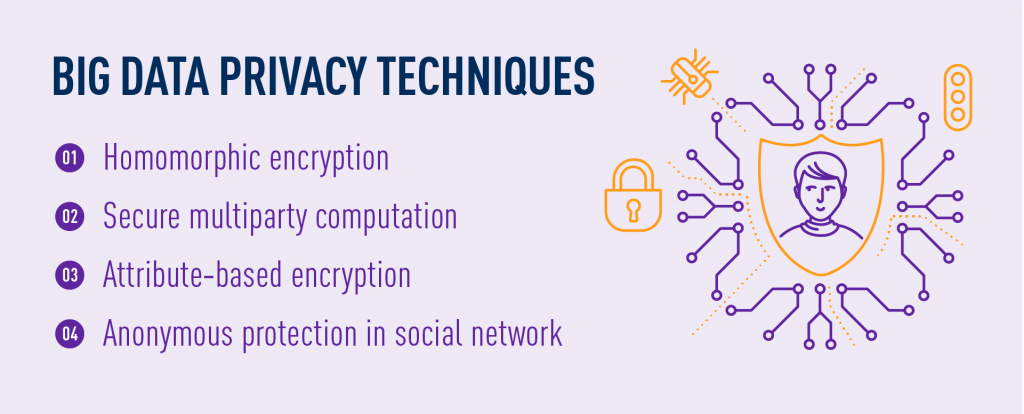
Homomorphic encryption
The concept of homomorphic encryption means that the data can operate with no influence on computing results without decrypting the ciphertexts.
Homomorphic encryption can be classified into two types:
- Semi‐homomorphic encryption that supports partial operations, like addition or multiplication,
- Fully homomorphic encryption that supports the calculation of arbitrary polynomials.
In homomorphic encryption schemes, each noise is hard to control, so it shows a low efficiency in the cloud environment. That’s why homomorphic encryption can’t meet the requirements of some application systems. Fortunately, in recent few years, a few researchers have tried to build homomorphic applications for solving Big Data privacy problems in the cloud environment.
Secure multiparty computation
A secure multiparty computation scheme was first proposed to calculate a function in a distributed cloud environment with their private inputs. After these calculations, all honest parties are given the right calculation results, but nothing except their own inputs and results. Furthermore, even if there are dishonest parties, the results of honest parties are not to be influenced. Based on secure multiparty computation, many researchers have proposed novel schemes for applying different security directions.
A secure self‐protection data scheme states the owners of data have absolute control over the outsourced data in a third‐party cloud environment. They combine four technologies:
- Attribute‐based encryption for the security of access control,
- RSA for key management,
- Active data bundles to self‐protect the data,
- Autonomous mobile agent for traffic control.
Based on safety, the scheme also reduces the consumption of data dissemination for cloud services.
Attribute‐based encryption
Attribute-based encryption has become the most appropriate and popular core encryption technique for secure access to data, particularly suitable for cloud computing. Different from ID‐based encryption, data owners label both keys and ciphertexts with the set of attributes. Then, users are required to prove the authority of legal users for these attributes. Only after that data is downloaded and decrypted by matching the characteristics of key and ciphertext.
Traditional attribute-based encryption is categorized into two types,
- Namely, ciphertext‐policy attribute-based encryption, in which the access protocols are embedded with homologous ciphertexts,
- Key‐policy attribute-based encryption, in which the access protocols are related to users’ private keys.
Furthermore, attribute-based encryption can be classified into two more categories, including single authority-based and multiple authorities-based. For the first one, all attributes are supervised by only one authoritative attribute. For the second one, multiauthority attributes monitor the properties of disjoint sets.
Anonymous protection in social network
Social network is one of the main reasons we worry about Big Data privacy. Different from schemes, user data in social networks is typically stored and managed in a graphical scheme. Hence, its anonymous protection is very different from structured data. Typical data protection needs in social networks are anonymous user identities and attributes. Hence, the latest popular anonymous social networks hide information and relationship between the users when messages are sent.
Researchers divide two types of schemes to protect security and privacy in social networks:
- Clustering-based methods, in which the nodes in the graph structure are segmented and clustered first according to attributes and then replaced by some supernodes; the detailed information is hidden so that the anonymity of users is realized; the method changes the semantics of the original social graph at the expense of the availability of data,
- Graph modification-based methods consider the additions and deletions of edges in a graph; the method of random additions and deletions of exchange edges can effectively achieve the desired level of anonymity; the key problem of this method is that the random increase in noise is too rare, and there is a lack of edge protection.
Best practices for keeping privacy in the age of Big Data
Companies should do whatever they can to protect Big Data security. Here we’re going to list some of the best practices for maintaining privacy in the age of Big Data.
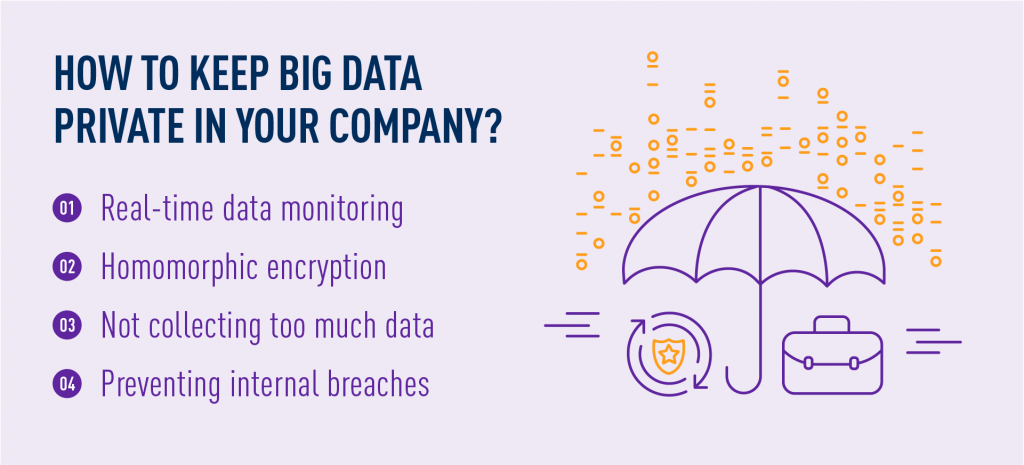
Real-time data monitoring
A data breach and other Big Data privacy issues can happen at any moment. Therefore, companies should find a solution that monitors their data in real time. This way, they’ll be aware of a problem as soon as it happens and can resolve it right away.
Implement homomorphic encryption
Homomorphic encryption allows users to compute data without decrypting it first. This form of encryption should be implemented to store and process data in the cloud to prevent organizations from revealing private information to outside vendors.
Avoid collecting too much data
Only absolutely necessary data should be collected. Companies may not need the Social Security numbers of their customers; customer login usernames and passwords may only be necessary. That’s why companies should consider deleting personal information that is not needed to best protect customer data privacy.
Prevent internal threats
Companies are also exposed to internal privacy risks from angry or simply uninformed employees. Therefore, it’s essential to educate all employees on best practices for ensuring Big Data privacy like frequent password changes and logging off computers.
TASIL – secure Big Data monetization platform
Data privacy is a big concern for companies that use Big Data. Big Data analytics ensures the ability to know so much about customers that it might scare us. That’s why the proper tools are needed.
TASIL is a secure solution that provides best-in-class features for digital information management. With our platform, you can process any data easily, collaboratively, and securely. Monetizing your mobile data while also preserving your customers’ privacy lies at the core of our solution.