Insights
- category:
Reducing customer churn with Big Data analytics tools
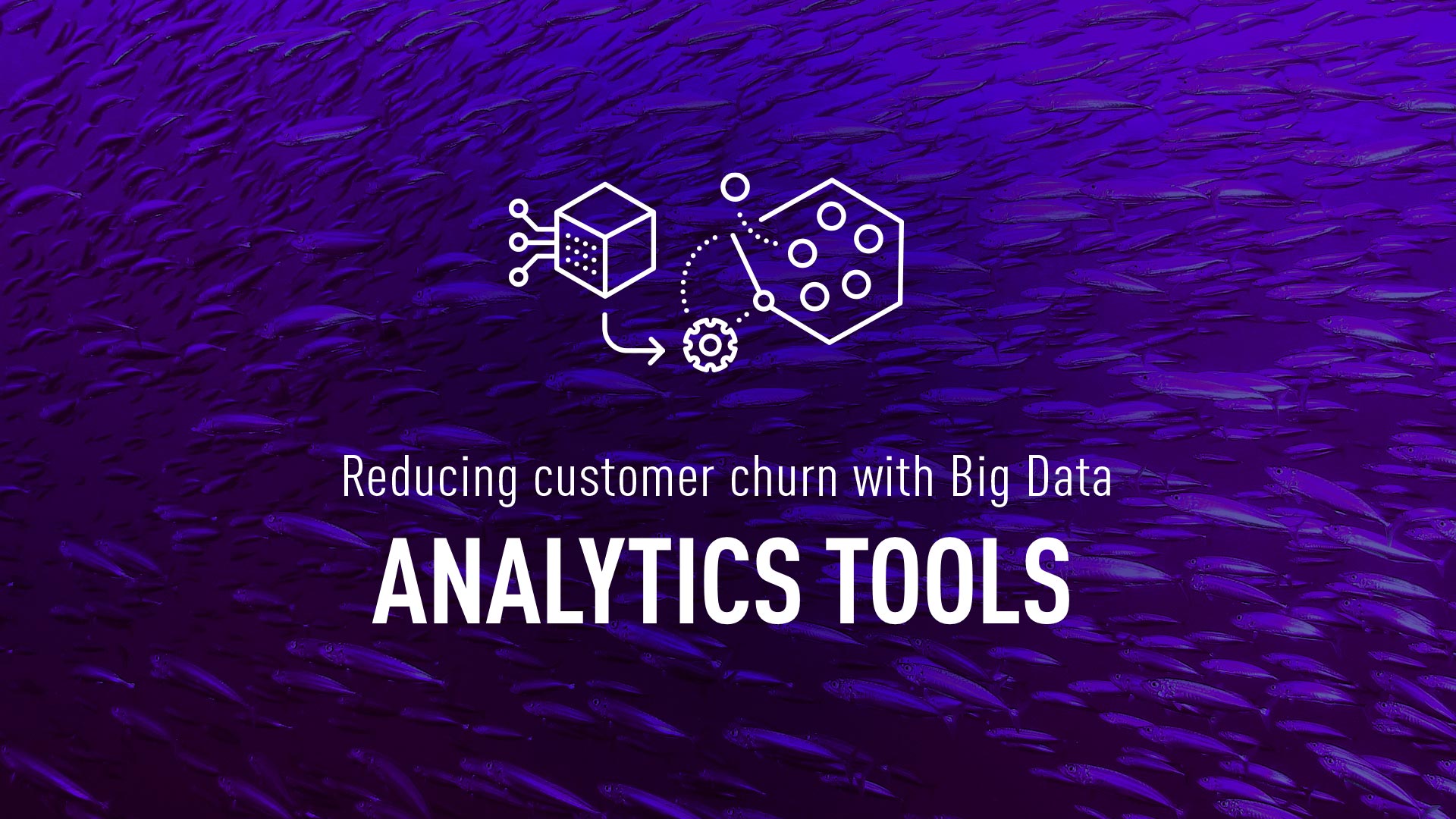
Thanks to large amounts of collected data, telecoms are in a unique position to understand their customers. Analyzing this data can help them deliver services perfectly adjusted to customers’ needs. But there is still one threat for telecoms, and that is customer churn. Using Big Data analytics tools can help reduce it while also gaining a significant competitive advantage.
What is customer churn?
Customer churn simply means losing customers. Some of the churned customers turn to a competitor, while some of them might simply leave the service forever. The company’s churn rate can be calculated by dividing the number of lost customers by the number of customers from the beginning of a selected period.
Customer churn is the percentage of customers that stopped using your company's product or service during a certain timeframe.
Customer churn is hard to predict and even harder to prevent. The enjoyment of service is a subjective experience that varies from customer to customer, even under the same circumstances. This is especially important for consumer services, like telecommunication or banking, because in these industries customer churn is particularly difficult to predict. Business companies tend to use a more rational analysis when deciding on renewing or cancelling service.
However, there are a few solutions that can help with reducing customer churn. Using Big Data analytics tools, segmentation, machine learning, or AI-based algorithms are a few examples.
How to reduce customer churn with Big Data analytics tools?
It’s a lot more expensive to acquire new customers than to retain existing ones. To make sure customers stay with you, you should focus on excellent customer service, attractive offers, effective personalization, rewards, and predicting future behaviour. Also, there are a few Big Data analytics tools that might help reduce customer churn and grow your customer base.
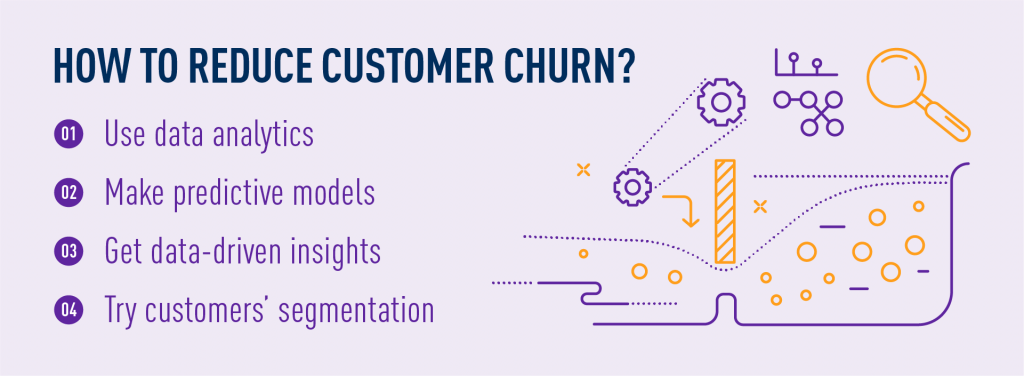
Use data analytics
Data analytics techniques can be used to control churn. Many companies don’t have a clear and sophisticated marketing strategy to embed data analytics. Taking an integrative approach and using analytics as a key strategic tool of growth can lead to accomplishing the desired results. Lifetime Value (LTV) analytics or A/B campaign management analytics are good examples.
LTV analytics estimates how much value a customer can bring to the company. It also prioritizes who needs to be targeted, indicates who can leave the company, or who needs to be treated with special attention. The A/B campaign management analytics observes pre- and post-campaigns sales and customers’ responses.
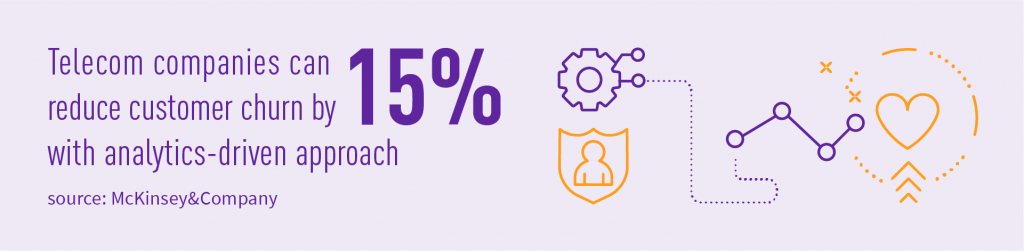
Both of these techniques allow companies to gather valuable insights that can be used to adjust their growth plan. Data analytics helps evaluate which customers need to be targeted, how many of them need personalized offers, or how much money the company should put in advertising.
Make predictive models
Machine learning and AI-based algorithms focus on using statistics, mathematics, and probability to find relationships among variables. Such solutions can be applied to make predictions and to give significant outcomes – such as churns and retentions.
To create sophisticated customer churn prediction strategies, companies can use predictive analytics. Looking at past datasets helps with determining what customers may like or dislike. This way, companies are one step closer to reducing churn rates.
Sometimes, during creating predictive models, it isn’t easy to manage and analyze all variables simultaneously. For making valuable predictive models of customer churn, the best solution is to rely on machine learning algorithms. They can accurately and quickly uncover the reasons why customers are churning or why they still like a given product.
Get data-driven insights
Data-driven insights can be achieved by analyzing free-text responses, which exist in the open-ended survey questionnaires. The best way to do it is with text analytics solutions, which use sentiment analysis to determine customers’ complaints.
Machine learning and AI-based algorithms are used to automate the analysis of free-text feedback. Natural language processing and machine learning can simplify the procedures of getting insights from customer data.
Advanced analytics also enables us to carry out AI-driven predictive analytics through sophisticated techniques such as predictive behaviour modelling, which is a mathematically intensive technique that can accurately predict churn in a specific customer micro-segment. Another is customer retention analysis, which can indicate how many new customers will stay with a company over time.
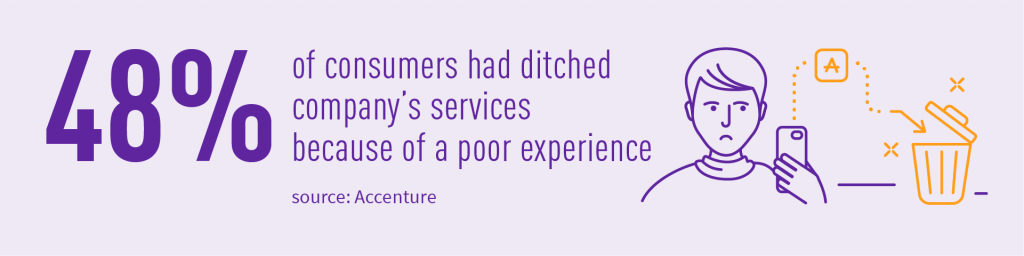
Thirdly, the Next Best Offer recommendation can predict what customers’ needs are before they realize them. NBO helps with putting together highly customized offers for your customers. Guiding customers to these offers at the right moment, using the most convenient channels for them, and at a price they will find most appealing is what companies can achieve with this mechanism.
Lastly, thanks to machine learning-based Natural Language Processing algorithms, it is possible to apply sentiment analysis. It can be used to analyze communication from various channels, such as social media comments, reviews, emails, and web chats. It has the power to identify positive, negative, or neutral emotions customers feel towards products and services. Such analysis is growing more and more sophisticated by the day. As for addressing customer churn, it can rapidly detect disgruntled customers, provide insights about their needs, and help win them over before they disappear.
Try customer segmentation
Finally, Big Data analytics tools enable customer segmentation. It allows companies to find out how each segment interacts with their product or services. The easiest way to do a segmentation is to analyze data about customers’ lifestyles, demographics, products purchased, their value, and the frequency of purchases. Such analysis, using the right type of variables, divides customers into groups of customers with similar interests and behaviours.
After performing customer segmentation, companies can choose the group that is driving the most revenue. Then, the whole marketing strategy can be adjusted to their needs. Personalized communications, special offers, or discounts – all these can improve churn prediction and increase retention for the most wanted customers.
Reducing customer churn with TASIL
TASIL uses Big Data analytics tools as the basis of its work. It can continuously gather data on customers and transform it into actionable insights. Thanks to these insights, we can focus our attention on bringing the best to our customers. Also, we can fully understand how consumers are using a specific product and how they make decisions accordingly. With the help of Big Data Analytics, we can find out how engaged consumers are with our services. Finally, by listening to and exceeding our customers’ needs, we can show them that we truly care.